April 24, 2025
Two years ago, BMW Group’s Hams Hall plant began an effort to centralize its data gathering infrastructure. This particular plant produces approximately 1.4 million components and assembles around 400,000 engines per year for a range of vehicles, generating a vast amount of data in the process.
That data, however, would often end up siloed, creating a serious operations oversight problem and the need, at one point, for internal teams to use more than 400 custom dashboards from 15 different IT systems.
So, BMW created a digital twin of the Hams Hall plant as a single source of truth accessible to all team members. Now, “everyone refers to the twin.”
The story of the Hams Hall plant reveals a significant challenge to leveraging digital twins: To get to an accurate digital twin - a single source of truth - typically involves integrating multiple sources of data of different types and access methods. Such a complex task is hindered by factors such as data management, accuracy, security, computing power, interoperability, and people.
Here’s a brief overview of 5 key challenges for digital twins:
DATA MANAGEMENT
As one source put it, data is the “lifeblood” of digital twins. Most organizations, however, don’t have the data management resources required for digital twins. The crux of the problem is that data is structured, shared, and accessed in fundamentally different ways across functions.
An industrial plant, for instance, might use hundreds of different software solutions and systems. Imagine the many assets, data types, data sources, protocols, etc.--all would need to be transformed to achieve data heterogeneity. In addition to the lack of data standardization, the high volume and frequency of data compound the technical challenges, even with advances in data storage and computing power.
The many issues around data - data availability, data recency, data complexity, data security, inaccurate or unsuitable data, etc. - aren’t unique to digital twins either. Data management is problematic for digitalization in general, a significant obstacle to digitizing processes and information. Moreover, an organization’s “data environment” is always shifting, as machines are replaced, sensors are added, and systems change.
You’ve probably heard of the “IT-OT divide:” Operations data is typically tied to specific applications. An OT system, for instance, might track machine temperature and format the data for immediate operational needs. IT systems, however, require standardized data decoupled from specific apps and rich in contextual information (e.g. machine temperature along with machine location, operational status, etc.)
For big data apps like digital twins, infrastructure must be in place to supply contextualized data in an analysis-ready format–a complex and expensive undertaking.
ACCURACY & SECURITY
Another data-related challenge is that of creating an accurate and reliable digital twin: There are so many factors affecting a single asset in a complex industrial environment like a factory. Valid testing with digital twins requires both clean data in a standardized format and the right data.
Consider the testing of an autonomous vehicle, a popular use case for digital twins in the automotive industry: As you can imagine, testing a self-driving car is difficult because it’s impossible to safely replicate every condition on the road.
Beyond a digital twin of the vehicle itself and all its components - aggregating visual and sensor data from multiple sources - you would have to make the simulated world physically accurate. That means accurately mimicking everything from varying light conditions and reflective car surfaces to road wear and bus stops.
All that to say, digital twins aren’t fool-proof. There’s risk involved in taking digital twin simulation-derived insights at face value today. Digital twins can also be a significant cybersecurity risk to an organization: The amount and oftentimes sensitive nature of data that goes into a digital twin makes it a tempting target for bad actors, leaving organizations vulnerable to theft of proprietary data, hijacking, etc.
COMPUTING POWER
Where there’s a lot of data, there’s a lot of processing, and the data associated with generating and maintaining digital twins is far greater than typical industry data in both size and complexity. Digital twins are an application of Artificial Intelligence (AI), and as such, require robust processing power.
Forget integrating vast amounts of data from multiple sources; imagine the power required to not only accurately represent the behavior of, say, an entire vehicle but also every component within and run complex simulations with it. Then add the need for fast processing, as we’re dealing with a continuous flow of real-time information from the physical world to maintain a true, live twin of the asset or system.
Most digital twin use cases leverage cloud computing (also edge) to provide the necessary computing power and storage, but highly detailed digital twins may need more advanced high-performance computing (HPC) setups. While some simulations can run on a workstation with a GPU, others require an entire data center. Naturally, the more intricate the twin, the greater the computational workload for it to function effectively.
INTEROPERABILITY
Like with any emerging technology, integrating digital twins into an organization’s existing infrastructure presents a major obstacle, especially in industries with legacy systems.
Digital twins require seamless communication between various devices, platforms and protocols, which is particularly problematic in the case of decades-old industrial facilities with decades-old equipment that’s unlikely to have the necessary IoT sensors. Even if an older piece of equipment has digital monitoring capabilities, that information is likely sent to siloed software somewhere.
Long-operating facilities also have a ton of archived data in unintelligent, unstructured formats that would need to be extracted and combined with information from legacy systems. Moreover, digital twins often require data from different areas of the business. Within a single company, for instance, there could be dozens of different 3D file formats, each one optimized for design, production, quality control, etc.
In that way, interoperability can be seen as an offshoot of the larger problem of data.
PEOPLE
As in most digital transformation projects, creating and deploying digital twins involves stakeholder management. Consider the case at the BMW plant: Such a comprehensive digital twin required coordinating efforts among multiple departments to ensure that only consistent, format-ready data is used and, critically, that the necessary data goes into the digital twin for it to serve every function of the value chain within the factory.
This involves clear communication, a willingness to share data, and not getting distracted. The more system-spanning the digital twin, the more likely key stakeholders will get pulled away to work on other projects. There’s also the usual resistance to change–the need to get users to trust the insights derived from digital twins.
Last but not least is a skills shortage: There’s a shortage of engineers with the domain knowledge and AI skills to build and maintain enterprise-level digital twins, adding to the upfront costs of implementation today.
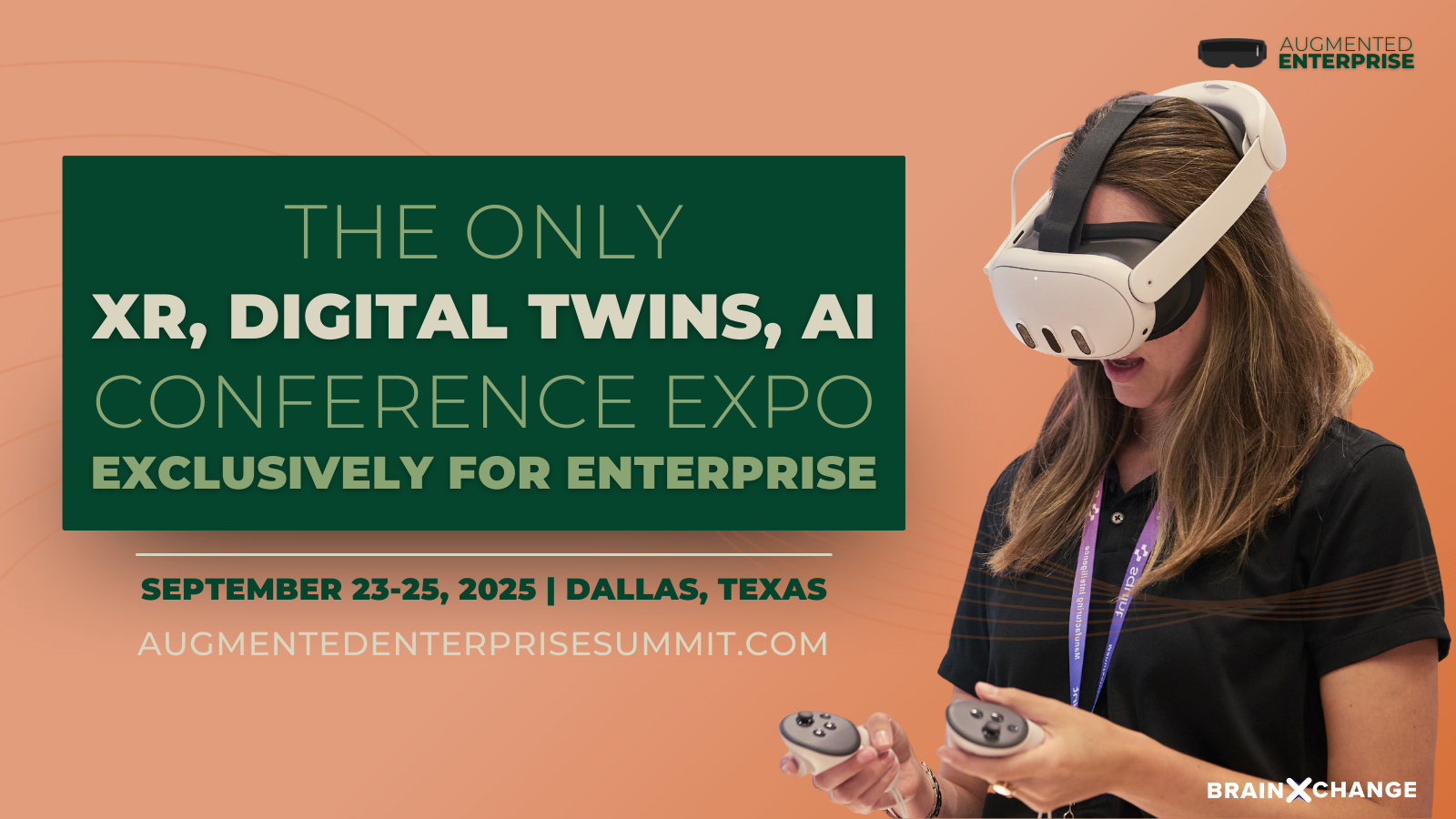